Making Datasets More Representative for More Equitable Artificial Intelligence
13 July 2020As with many things in life, you get out of them what you put into them. It turns out the same is true for artificial intelligence, or AI. AI is powered by datasets that are labeled or annotated by humans. For example, creating software that translates spoken language into text requires extensive human input and labeling. These labeled datasets are then augmented by computers and used to “train” machine learning models. Yet while machine learning has delivered incredible benefits such as the automatic speech recognition (ASR) that enables me to speak over the phone with my grandmother who is hearing impaired—AI is only as good as the data we humans put into it.
The problem is, there are vast gaps in the data available to train computer models. I’m lucky to communicate with my grandmother in English. ASR systems for many non-European languages are far less developed. Ask a non-native speaker of English how easy it is to get help over the phone using an automated speech recognition system, and you’ll get a sense of how far we have to go.
Everything from whole populations, to data from entire regions, are missing from the datasets used to power AI. This can lead to woefully inadequate tools or even inaccurate results. For example, skin cancer detection models enable doctors to more efficiently diagnose disease and get patients into treatment. Unfortunately, most of these models are trained using images of lighter complexioned skin, so they are not as effective for patients with black or brown skin. This can have life and death consequences.
In many cases, data required to build AI applications for real-world problems doesn’t exist. And where it does exist, it’s often outdated, missing key information, or not representative of underserved populations – leading to biases and decreased accuracy. Machine learning tools then “learn” these biases. This can harm or exclude people of color, women, and other marginalized populations.
A lacuna is a gap, a blank space, or a missing part. Data scientists, funders, and social entrepreneurs around the world have recognized the missing information as a lacuna in labeled datasets and are committed to closing these gaps in order to reduce bias and improve accuracy. The Rockefeller Foundation, Google.org, and Canada’s International Development Research Centre have partnered with these technical experts, affected stakeholders, and end users to found Lacuna Fund, an initiative that will mobilize resources to fund open source, labeled datasets to solve urgent problems in low- and middle-income contexts globally. Others are welcome to contribute too, either to the pooled fund or to targeted Requests for Proposals (RFPs). The German development agency (GIZ), on behalf of the German Federal Ministry for Economic Cooperation and Development (BMZ), is providing financial support and technical assistance for an RFP on natural language processing.
Lacuna Fund’s first two RFPs focus on data collection and labeling for agriculture and language portfolios in Africa. The resulting datasets will make it possible to provide smallholder farmers with important data about crop and livestock selection and management that could increase yields. In the language domain, new and expanded datasets will enable governments to communicate with people in their own languages in emergency situations. The Fund is also issuing a Request for Information to better understand the labeled data needs of healthcare professionals working on COVID-19 and other respiratory illnesses.
The first RFP on agricultural datasets in sub-Saharan Africa is open until 3 September 2020. Read more about the RFP and sign up to receive notifications for upcoming opportunities here.
Meridian Institute serves as the secretariat and fiscal agent for Lacuna Fund. In these roles, we provide backbone support, leveraging core strengths in facilitation, implementation, and philanthropic support. We facilitate the Fund’s Steering Committee and Technical Advisory Panels, helping funders, machine learning experts, and end users reach agreement on guiding principles, the focus of funding calls, proposal selection, and fund operations. Meridian also manages the RFP process and disbursement of funds. We are working to establish a future secretariat for the Lacuna Fund within an institution in the Global South.
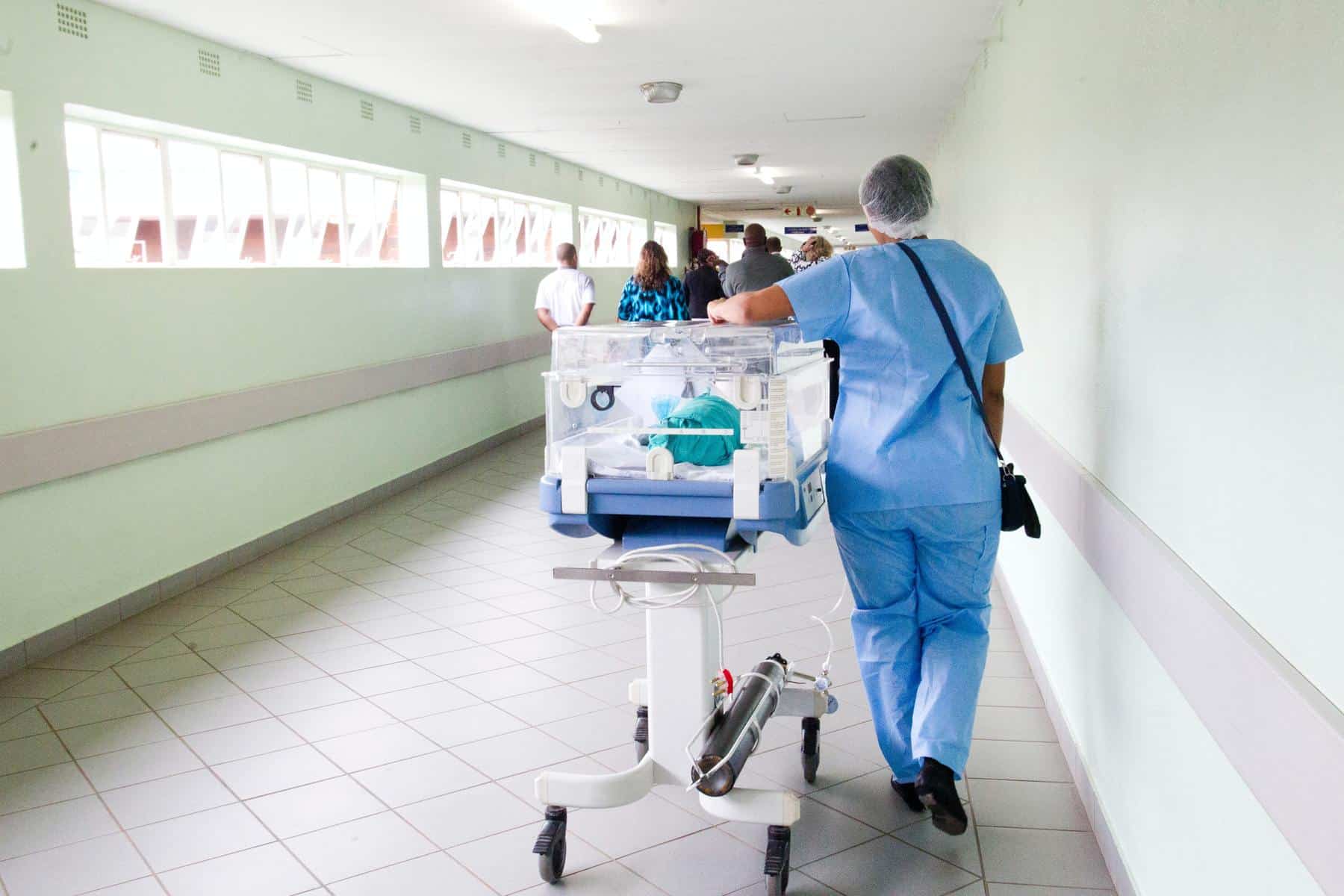
Learn more about Lacuna Fund
Check out how the Fund is helping put the benefits of machine learning within reach of data scientists, researchers, and social entrepreneurs worldwide.